Single imputation methods
Single imputation denotes that the missing value is replaced by a value. In this method the sample size is retrieved. However, the imputed values are assumed to be the real values that would have been observed when the data would have been complete. When we have missing data, this is never the case. We can never be completely certain about imputed values. Therefore this missing data uncertaintly should be incorporated as is done in multiple imputation.
Mean imputation
Mean imputation is a method in which the missing value on a certain variable is replaced by the mean of the available cases. This method maintains the sample size and is easy to use, but the variability in the data is reduced, so the standard deviations and the variance estimates tend to be underestimated. The magnitude of the covariances and correlation also decreases by restricting the variability and this method often causes biased estimates, irrespective of the underlying missing data mechanism (Enders, 2010; Eekhout et al, 2013). In the example below you can see the relation between x and y when the mean value is imputed for the missing values on y.
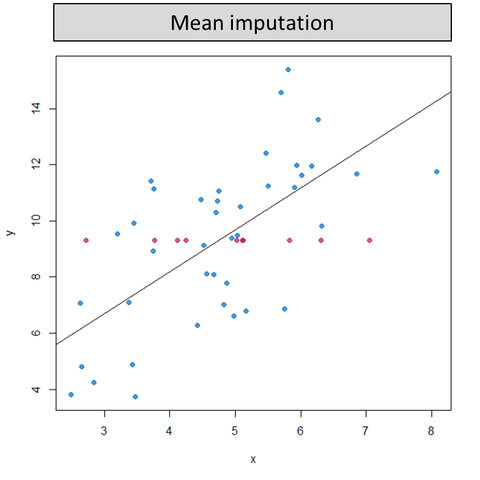
For missings on multi-item questionnaires, mean imputation can be applied at the item level. One option is to impute the missing item scores with the item mean for each item. In that case the average of the respondents with observed scores for each item is computed and that average value is imputed for respondents with a missing score. Another option is to impute the person mean. In that method the average of the observed item scores for each respondent is computed and that average is imputed for the item scores that are missing for that respondent. This option is also called average of the available items. Both these methods result in biased analysis results, especially when missing data are not MCAR (Eekhout et al. 2013). Nevertheless, these methods are often advised in questionnaire manuals.
Another method, that combines item mean imputation and person mean imputation is two-way imputation. In this method the imputed value is calculated by adding the person mean to the item mean and subtract the overall mean from that score (van Ginkel et al. 2010).
Regression imputation
In single regression imputation the imputed value is predicted from a regression equation. For this method the information in the complete observations is used to predict the values of the missing observations. Regression assumes that the imputed values fall directly on a regression line with a nonzero slope, so it implies a correlation of 1 between the predictors and the missing outcome variable. Opposing the mean substitution method, regression imputation will overestimate the correlations, however, the variances and covariances are underestimated.
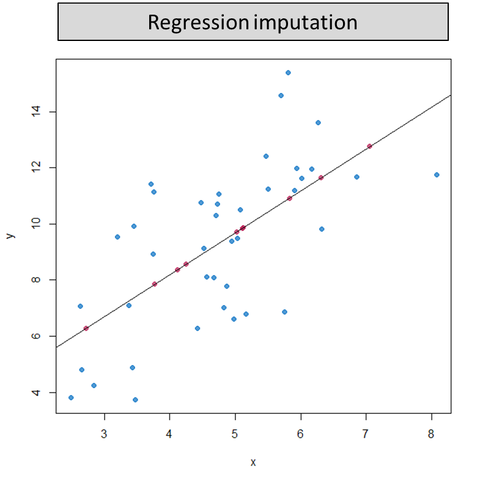
Stochastic regression imputation aims to reduce the bias by an extra step of augmenting each predicted score with a residual term. This residual term is normally distributed with a mean of zero and a variance equal to the residual variance from the regression of the predictor on the outcome. As you can see in the video below, the error that is added to the predicted value from the regression equation is drawn from a normal distribution. This way the variability in the data is preserved and parameter estimates are unbiased with MAR data. However, the standard error tends to be underestimated, because the uncertainty about the imputed values is not included, which increases the risk of type I errors (Enders, 2010).
Matching methods
Hot-deck imputation is a technique where non-respondents are matched to resembling respondents and the missing value is imputed with the score of that similar respondent (Roth, 1994). Two hot-deck approaches are the distance function approach and the pattern matching approach. The distance function approach, or nearest neighbor approach, imputes the missing value with the score of the case with the smallest squared distance statistic to the case with the missing value. The matching pattern method is more common, where the sample is stratified in separate homogenous groups. The imputed value for the missing case is randomly drawn from cases in the same group (Fox-Wasylyshyn & El-Masri, 2005). Hot-deck imputation replaces the missing data by realistic scores that preserve the variable distribution. However it underestimates the standard errors and the variability (Roth, 1994). Hot-deck imputation is especially common in survey research (Little & Rubin, 2002).
Last observation caried forward
The last value carried forward method is specific to longitudinal designs. This technique imputes the missing value with the last observation of the individual. This method makes the assumption that the observation of the individual has not changed at all since the last measured observation, which is mostly unrealistic (Wood, White & Thompson, 2004).